
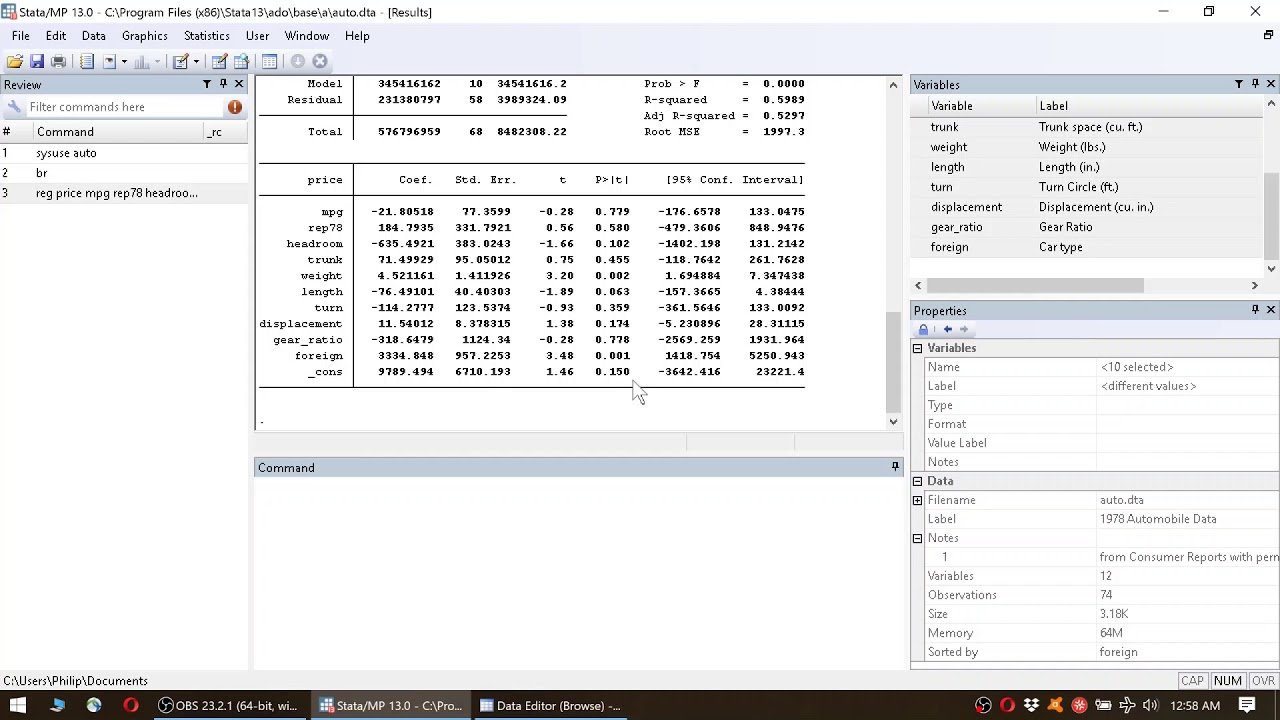
The value of z- statistic corresponding to all coefficients is greater than 2 in absolute value, thus we reject the null hypothesis that the corresponding beta coefficient is 0. Lower standard errors corresponding to all the coefficients indicate that the estimated values are close to the actual values. The standard error value of 0.0083 corresponding to variable EDU indicates that when repeated samples are drawn, 95% of times the estimated years of education will lie within 0.0166 (2 times standard error, which is 0.0083) of true years of education. The standard errors in output table indicate dispersion in estimated values around the average, in the distribution of the variable being considered. Thus, individual with absolutely no education and experience derives an hourly wage equals to antilog (0.5941). The estimated value of constant term can be interpreted as the minimum hourly wage earnings in dollar obtained by an individual with no education and experience. Similarly, the slope coefficient corresponding to potential years of experience (EX) implies that one unit change in potential years of experience leads to 1.17% change in average hourly earnings in the same direction ( indicated by a positive sign with the coefficient), given all other variables are kept unchanged. The slope coefficient corresponding to years of education (EDU) implies that one unit change in years of education leads to 9.64% change in average hourly earnings in same direction (indicated by a positive sign with the coefficient), given all other variables are kept unchanged. The STATA command for this problem is given below and the regression output obtained is as follows: Regress LNWAGE EDU EX Interpretation of Multiple Linear Regression Output from STATA Regress Independent Variable Dependent variable1 Dependent variable2 Dependent Variable3……. The command for STATA for conducting multiple linear regression in STATA is: Using ordinary least square method (OLS) of estimation, we regress LNWAGE on a constant, years of schooling and experience.

Years of education (EDU) and potential years of experience (EX) are the regressors. The natural logarithm of average hourly wage earning in dollar (LNWAGE) is the regressand,Ĭ refers to a constant term in the regression equation, Using STATA, we estimate the following equation: LNWAGE = C + β 1EDU + β 2EX What would be the problem of including AGE as an additional regressor? MULTIPLE LINEAR REGRESSION IN STATA (Only Quantitative Independent Variables) What do the slope coefficients on EDU and EX measure? Intuitively, age may affect as well. Using OLS, let us estimate the parameters in a simple model where LNWAGE is regressed on a constant, years of schooling (EDU), and experience (EX) and report these along their estimated standard errors.
